Dobb·E
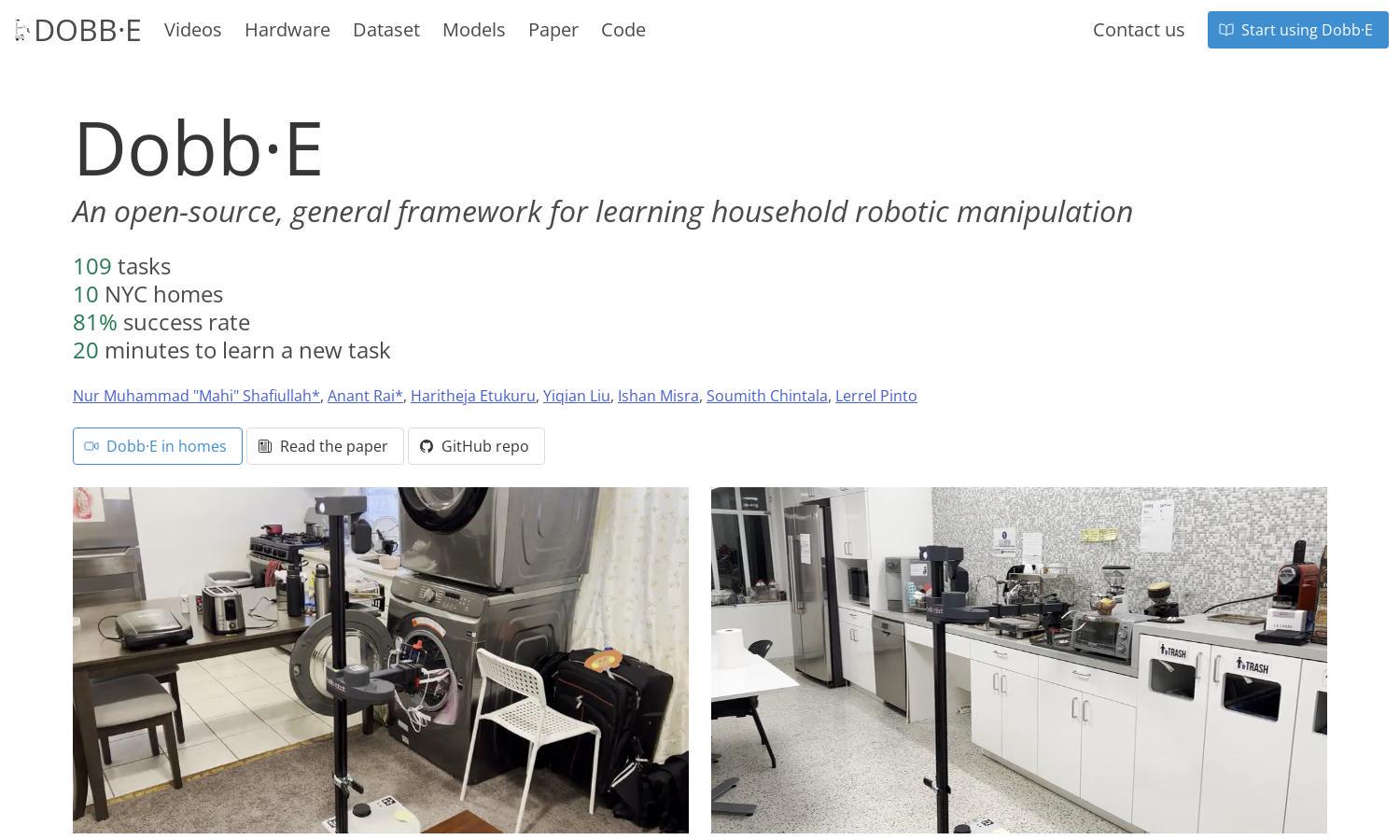
About Dobb·E
Dobb·E is a cutting-edge platform designed for home robotics. It allows users to train robots in household tasks with just 20 minutes of demonstration. Utilizing the Stick tool, Dobb·E collects data, trains its model, and adapts to new environments, significantly simplifying robotic learning for efficient household integration.
Dobb·E’s pricing structure offers free access to its open-source software and resources. Users can utilize the innovative framework without subscription costs, providing exceptional value as they train robots for household tasks. The benefit of this accessible model encourages wider experimentation and adoption in the home robotics space.
Dobb·E features an intuitive interface designed for easy navigation. Users can seamlessly shift from data collection to modeling, aided by clear instructions and effective design. This user-friendly layout enhances the experience of learning robotic tasks, making Dobb·E a practical choice for anyone interested in home automation.
How Dobb·E works
To interact with Dobb·E, users begin by using the Stick tool to demonstrate tasks in their home. Data is collected over five minutes, which is then used to train the Home Pretrained Representations (HPR) model. After that, Dobb·E adapts the model to solve novel tasks in just 15 minutes, achieving high success rates and simplifying robot training.
Key Features for Dobb·E
Imitation Learning Framework
Dobb·E's imitation learning framework allows users to teach robots household tasks rapidly. By leveraging the Stick for data collection, Dobb·E efficiently trains models, drastically reducing the time and effort needed for robots to learn and perform various domestic duties, creating a versatile home assistant.
Homes of New York Dataset
The Homes of New York (HoNY) dataset is a comprehensive repository created by Dobb·E, containing over 13 hours of household interaction data. This dataset enhances Dobb·E’s training capabilities and aids in developing more effective robotic assistance solutions tailored to diverse home environments.
Home Pretrained Representations (HPR)
Home Pretrained Representations (HPR) is a core feature of Dobb·E, providing a foundation for swift robot learning. Pretrained on the HoNY dataset, HPR allows robots to adapt to new tasks with minimal demonstrations, significantly increasing their operational efficiency in varied home settings.
You may also like:
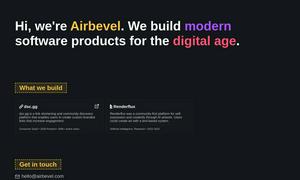
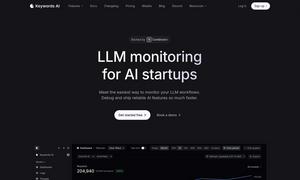
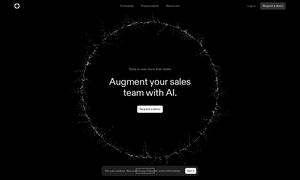