Velvet
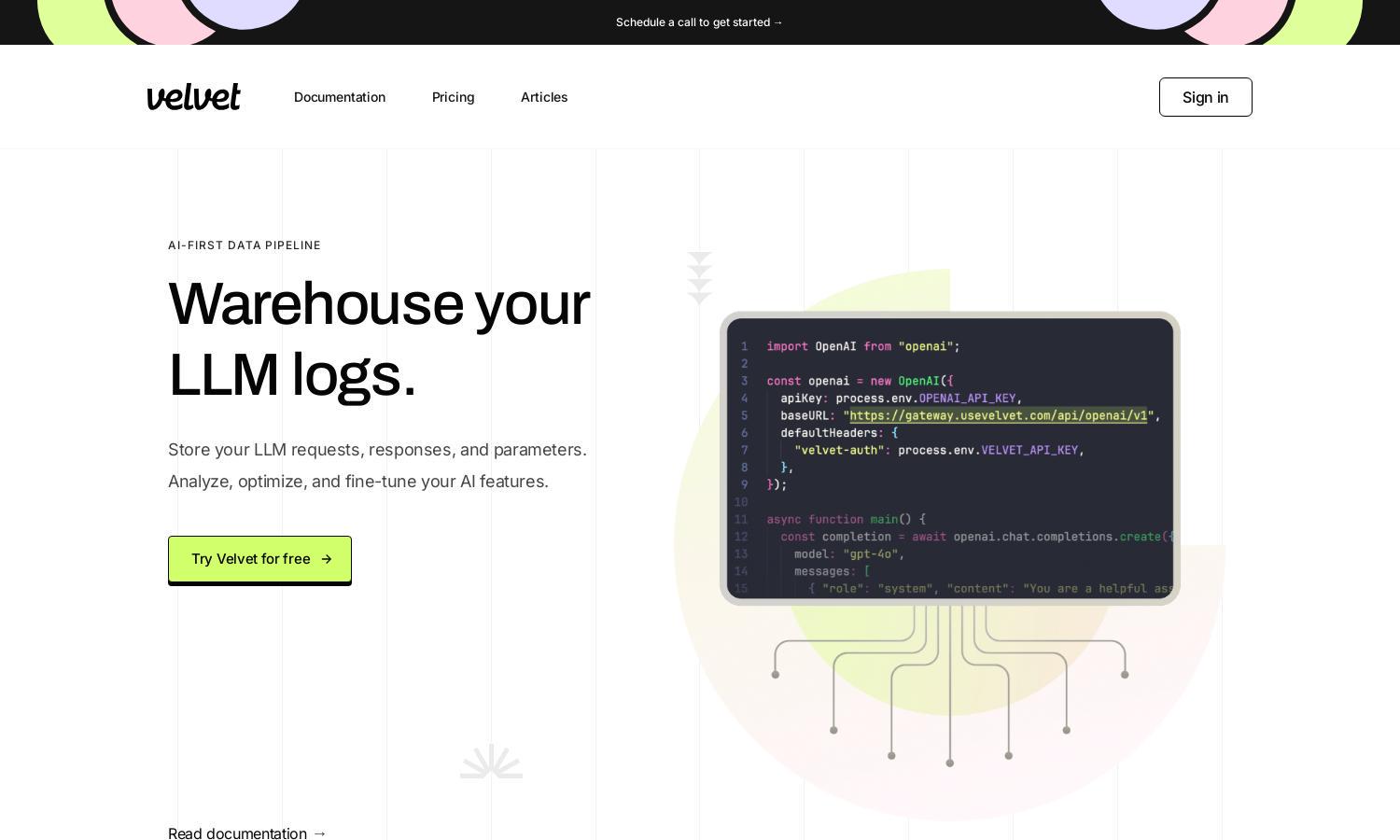
About Velvet
Velvet streamlines AI operations for engineers by providing a powerful gateway to log and analyze requests. With simple integration, users can explore their data, run experiments, and optimize features while gaining deep insights into usage and costs, making Velvet an essential tool for modern engineering teams.
Velvet offers a free basic plan allowing up to 10,000 requests per month. Users can explore additional features by upgrading their accounts for extended capabilities and support, making it accessible for teams looking to enhance their AI functionalities while managing costs effectively.
Velvet's user interface is designed for ease of use, enabling seamless navigation through features like logging, analysis, and experimentation. Its intuitive layout fosters efficient interactions, ensuring users can quickly access insights and optimize their AI operations, creating a productive environment.
How Velvet works
To get started with Velvet, users first create an account on the website. Following registration, they read the comprehensive documentation to understand the platform's capabilities. By setting the appropriate baseURL, users connect their database to Velvet’s analysis tools, allowing them to monitor and optimize AI feature usage effortlessly.
Key Features for Velvet
Logging Requests
Velvet's logging feature warehouses every OpenAI and Anthropic API request, providing detailed insights into usage. This unique capability empowers engineers to evaluate performance, identify costs, and make informed decisions while optimizing their AI workflows, enhancing the overall efficiency of their engineering processes.
Optimized Caching
With Velvet's optimized caching feature, engineers can dramatically reduce costs and latency in processing AI requests. This unique offering not only streamlines operations but also enhances the user experience by ensuring rapid response times, thereby improving overall efficiency when working with AI models.
Experimentation Framework
Velvet's experimentation framework allows users to run controlled tests on various datasets, optimizing AI outputs at scale. This distinct feature supports engineers in fine-tuning models and prompts, facilitating continuous improvement in performance and delivering more accurate results for their applications.
You may also like:
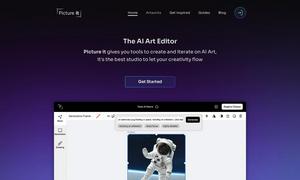
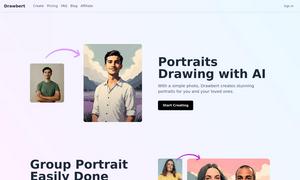